Source: The Conversation (Au and NZ) – By Arianna Olivelli, Research Affiliate, CSIRO
It’s estimated about two million tonnes of plastics enter the oceans from rivers each year. But our waterways aren’t just conveyor belts transporting land waste to the oceans: they also capture and retain litter.
Currently, the most common method for monitoring litter relies on humans conducting on-ground visual counts. This process is labour-intensive and makes it difficult to monitor many locations simultaneously or over extended periods.
As part of CSIRO’s research to end plastic waste, we’ve been developing an efficient and scalable environmental monitoring system using artificial intelligence (AI).
The system, part of a larger pilot with the City of Hobart, uses AI-based image recognition to track litter in waterways.
Global insights help build a reliable model
The technology is underpinned by two branches of AI: computer vision and deep learning. Computer vision involves training computers to understand and interpret images and videos, whereas deep learning imitates how our brains process data.Drawing on these capabilities, we worked in partnership with Microsoft (using its Azure cloud computing services) to develop an automated system for monitoring river litter.
We have been detecting and classifying items floating on the surface of Hobart’s stormwater channels, the River Thames in the UK and the Buriganga River in Bangladesh.
We’ve remotely analysed the amount of litter, the type of litter and how this changes across locations.
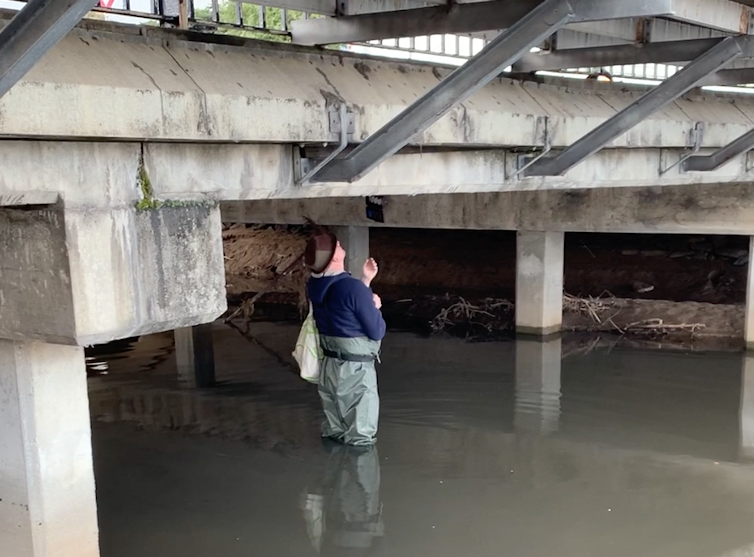
Major damage from food packaging and bottles
Our work relies heavily on two applications of computer vision. These are “object detection” and “image classification”.
Object detection specifies the location of a particular object in an image and assigns it a label. Image classification assigns one or more labels to the image as a whole.
Before either of these models can be applied reliably, however, they have to be trained, tested and validated using a large number of labelled images. For this, we drew from our footage of river litter collected from Hobart, London and Dhaka.
Our dataset now contains more than 6,100 images with 14,500 individual items. The items are labelled across more than 30 categories including plastic bottles, packaging, beverage cans, paper and plastic cups.
Our data revealed food packaging, beverage bottles and cups were by far the most frequently spotted litter items across all three countries.
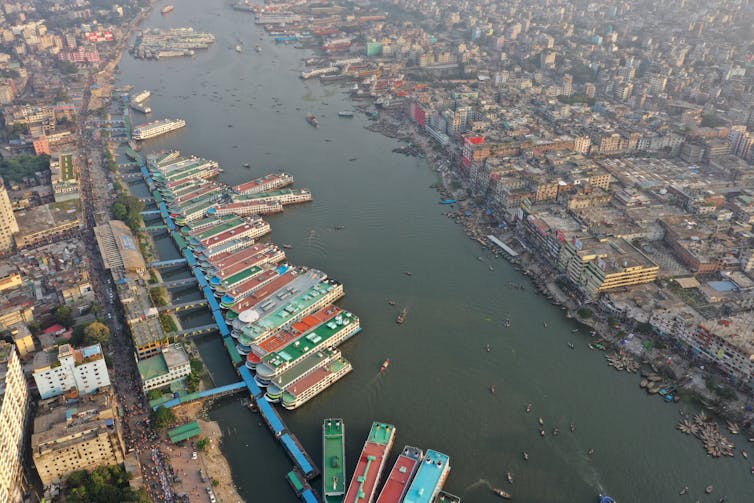
Fake images aren’t always harmful
To build a well-performing machine learning model, we needed a balanced set of training images featuring all item categories — even if certain categories are more frequent in real life.
Introducing synthetic (computer generated) images to our dataset was a game changer.
These images were generated by Microsoft’s synthetics team based in Seattle. They rendered various objects and superimposed them over backgrounds obtained from our field photos.
Once the digital objects were created, the superimposition process was automatic. Thus, the team managed to produce thousands of synthetic pictures over just a few weeks, rapidly expanding our training dataset.
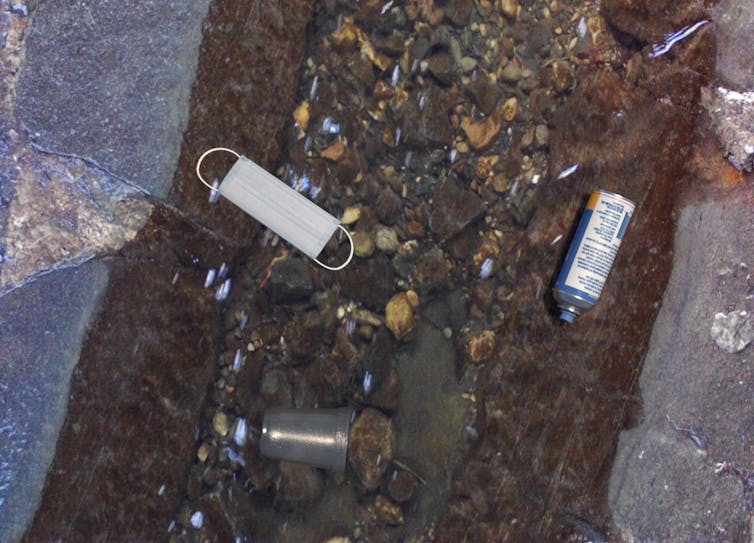
How are objects identified?
There are a few steps by which our system identifies litter objects in photos. First, the photos are all scored against a single-label (“trash”) object detector. This identifies items of litter in the frame and stores their coordinates as annotations.
These coordinates are then used to isolate the items and score them against an image classifier which includes all the litter categories.
Finally, the model presents the category it thinks the item most likely belongs to, along with a suggested probability for how accurate this guess is.
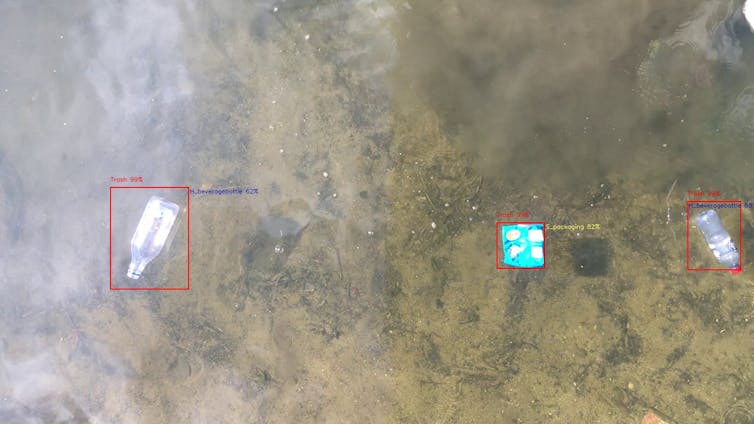
An AI-driven approach to litter management allows a quicker response than a manual system. But when it comes to litter, the major challenge lies in creating a model that can account for millions of different shapes, colours and sizes.
Read more: As cities grow, the Internet of Things can help us get on top of the waste crisis
We wanted to build a flexible model that could be transferred to new locations and across different river settings, including smaller streams (such as Hobart’s stormwater system) and large urban rivers (such as the River Thames or the Buriganga River).
This way, rather than building new models for each location, we only have to deploy more cameras. Data retrieved could help identify litter hot spots, implement better waste-related policies and improve waste management methods to make them safer, smarter and relatively cheaper.
Keeping an eye on Hobart’s litter
We’ve also been collaborating with the City of Hobart to develop an autonomous sensor network to monitor gross pollutant traps, such as floating barriers or litter socks.
These structures, integrated into Hobart’s stormwater drainage system, are supposed to prevent solid waste such as cans, bottles, tree branches and leaves from reaching the estuary and ocean.
We currently have a network of sensors and six cameras installed under bridges tracking litter in the traps. The system can inform an operator when a trap requires emptying, or other maintenance.
Once in full use, the technology will provide almost real-time monitoring of litter around Hobart, assisting efforts to reduce environmental harm caused by stagnant and potentially hazardous waste lost to the environment.
Read more: How sensors and big data can help cut food wastage
– ref. From Hobart, to London, to Dhaka: using cameras and AI to build an automatic litter detection system – https://theconversation.com/from-hobart-to-london-to-dhaka-using-cameras-and-ai-to-build-an-automatic-litter-detection-system-150950